RENAISSANCE, a generative machine learning framework capable of efficiently measuring large-scale kinetic model parameters.
MOLECULAR & COMPUTATIONAL BIOLOGY
9/2/2024
Researchers at the École Polytechnique Fédérale de Lausanne have successfully developed RENAISSANCE, a generative machine learning framework capable of efficiently and dynamically measuring large-scale kinetic model parameters to align with experimental observations. RENAISSANCE integrates various omics data and other relevant information, such as extracellular medium composition, physicochemical data, and domain expertise, to accurately characterize intracellular metabolic states. In a study published in Nature Catalysis in August 2024, RENAISSANCE demonstrated its ability to precisely reflect the metabolic behaviour of Escherichia coli even when faced with diverse variables such as genetic changes and environmental conditions. RENAISSANCE also showed proficiency in estimating missing kinetic parameters and reconciling them with existing experimental data, significantly reducing uncertainty in parameters. Despite some limitations, RENAISSANCE offers a promising tool for researchers studying metabolic changes, supporting biotechnology development for various future applications.
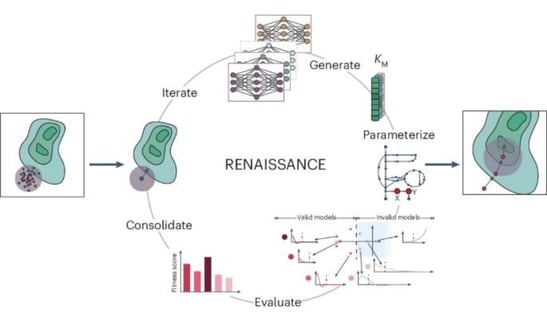
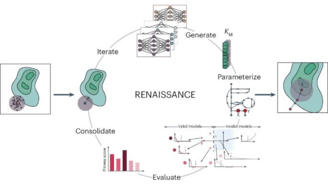